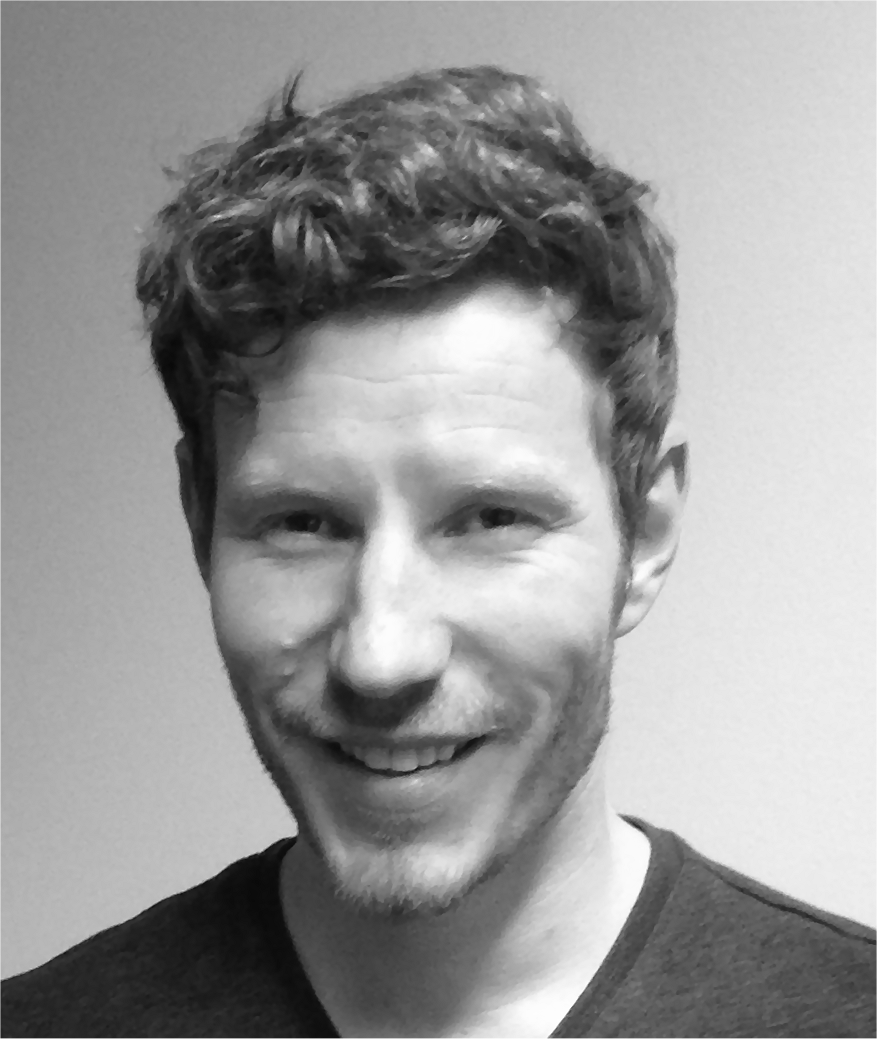
2019-04-05
10:15 - 11:15
Visual Computing Forum
Jürgen Bernard is Post-doc at the department of Computer Science, TU Darmstadt, Germany. He is leading the Visual-Interactive Machine Learning research group in the Interactive Graphics Systems Group (GRIS). He was with Fraunhofer IGD when he received is PhD degree in 2015, entitled “Exploratory Search in Time-Oriented Primary Data”. In 2016, his work was awarded with the Hugo-Geiger Prize for excellent PhD theses, granted by the Fraunhofer Foundation. His research focus is in information visualization, visual analytics, interactive machine learning, interactive data science, human-computer interaction, and user-centered design. In his design studies, he has collaborated with domain experts from multiple application fields, including medical health care, climate and weather research, digital libraries, digital humanities, alternative energy resources, finance or sensor networks, human motion analysis and animation, and data-driven patient-centered health. In 2017, J. Bernard received the Dirk Bartz Prize for Visual Computing in Medicine, granted by the Eurographics Association (EG). His data-centered focus is on multivariate data, multi-modal data, and time-oriented data. Many of his visual-interactive solutions include and combine data science techniques such as cluster analysis, classification, dimensionality reduction, similarity search, active learning, interactive data labeling, or other approaches related to information retrieval, data mining, and machine learning.
Abstract:
Machine Learning is a key technology in the era of data-centered and data science applications. Effective Machine Learning models have been trained in the past, that ease our everyday life or gain other types of promising benefits. However, the human-centered perspective of the Machine Learning process is still less understood. Today, in many cases humans still do not fully understand how analytical models have been trained, why models predict a particular output for a given input instance, or whether predictions of these models always reflect humans’ notion of judgement in a meaningful way. In most cases, end users rely on the expertise of data scientists who need to act as a sort of mediator in the Machine Learning process. One can only hope that the information need and abilities of end users (domain knowledge, expertise, and individual preferences) are well reflected in existing data science solutions. This talk motivates the human-centered perspective of Machine Learning. The basic idea is to conflate the strengths of visualization, HCI, and algorithmic models to foster the human-centered Machine Learning process. Users will be able to play a more active role in the process and become an integral part of highly interactive and iterative training and validation cycles. I will demonstrate that visual analytics is a promising approach to address this endeavor. In two examples research directions, I will show how human-centered Machine Learning can be put into practice. The first example is the exploratory search in time-oriented data. I will demonstrate how the design and application of content-based exploration and search systems can be adopted to time-oriented data. The second example refers to “Visual-Interactive Labeling” (VIAL). I will demonstrate how the strengths of humans and algorithmic models can be conflated to foster the labeling of unknown data sets and to apply interactive machine learning.